Genetic Matcher
The GeneticMatcher can be used to optimize any function of the baseline covariates, both linear and non-linear. In this demo notebook, we show how to call the matcher in the PyBalance library, including an example of a non-linear balance function.
[1]:
import logging
logging.basicConfig(
format="%(levelname)-4s [%(filename)s:%(lineno)d] %(message)s",
level='INFO',
)
from pybalance.sim import generate_toy_dataset
from pybalance.utils import (
BetaBalance,
BetaSquaredBalance,
BetaXBalance,
BetaMaxBalance,
GammaBalance,
GammaSquaredBalance,
GammaXBalance,
GammaXTreeBalance,
MatchingData
)
from pybalance.genetic import GeneticMatcher, get_global_defaults
from pybalance.visualization import (
plot_numeric_features,
plot_categoric_features,
plot_binary_features,
plot_per_feature_loss,
)
time_limit = 300
[2]:
m = generate_toy_dataset()
m
[2]:
Headers Numeric:
['age', 'height', 'weight']
Headers Categoric:
['gender', 'haircolor', 'country', 'binary_0', 'binary_1', 'binary_2', 'binary_3']
Populations
['pool', 'target']
['age', 'height', 'weight']
Headers Categoric:
['gender', 'haircolor', 'country', 'binary_0', 'binary_1', 'binary_2', 'binary_3']
Populations
['pool', 'target']
age | height | weight | gender | haircolor | country | population | binary_0 | binary_1 | binary_2 | binary_3 | patient_id | |
---|---|---|---|---|---|---|---|---|---|---|---|---|
0 | 62.511573 | 190.229250 | 105.165097 | 0.0 | 2 | 3 | pool | 0 | 0 | 0 | 0 | 0 |
1 | 68.505065 | 161.121236 | 95.001474 | 0.0 | 1 | 1 | pool | 1 | 0 | 1 | 0 | 1 |
2 | 50.071384 | 162.325356 | 84.290576 | 1.0 | 0 | 5 | pool | 0 | 0 | 1 | 1 | 2 |
3 | 44.423692 | 150.948096 | 82.031381 | 1.0 | 2 | 2 | pool | 0 | 0 | 0 | 1 | 3 |
4 | 41.695052 | 132.952651 | 54.857540 | 0.0 | 1 | 3 | pool | 0 | 0 | 1 | 1 | 4 |
... | ... | ... | ... | ... | ... | ... | ... | ... | ... | ... | ... | ... |
995 | 21.474205 | 168.602546 | 70.342128 | 0.0 | 2 | 5 | target | 0 | 0 | 0 | 1 | 10995 |
996 | 40.643320 | 188.188724 | 61.611744 | 0.0 | 2 | 4 | target | 1 | 0 | 0 | 1 | 10996 |
997 | 29.472765 | 161.408162 | 57.214095 | 0.0 | 0 | 1 | target | 0 | 1 | 1 | 1 | 10997 |
998 | 41.291949 | 150.968833 | 91.270798 | 0.0 | 0 | 3 | target | 0 | 0 | 0 | 0 | 10998 |
999 | 67.530294 | 155.124741 | 56.196505 | 1.0 | 0 | 1 | target | 1 | 0 | 0 | 0 | 10999 |
11000 rows × 12 columns
Optimize Beta (Mean Absolute SMD)
[3]:
objective = beta = BetaBalance(m)
matcher = matcher_beta = GeneticMatcher(
matching_data = m,
objective = objective,
log_every = 1000,
n_generations = 5000,
time_limit = time_limit
)
matcher.get_params()
INFO [matcher.py:125] cpu
[3]:
{'objective': 'beta',
'candidate_population_size': 1000,
'n_candidate_populations': 1024,
'n_keep_best': 256,
'n_voting_populations': 256,
'n_mutation': 256,
'n_generations': 5000,
'n_iter_no_change': 100,
'time_limit': 300,
'max_batch_size_gb': 2,
'seed': 1234,
'verbose': True,
'log_every': 1000,
'initialization': {'benchmarks': {'propensity': 'include'},
'sampling': {'propensity': 1.0, 'uniform': 1.0}}}
[4]:
matcher_beta.match()
INFO [initialization.py:31] Optimizing balance with genetic algorithm ...
INFO [initialization.py:32] Initial balance scores:
INFO [initialization.py:37] beta: 0.233
INFO [initialization.py:38] Initializing candidate populations ...
INFO [initialization.py:86] Computing PROPENSITY 1-1 matching method ...
INFO [matcher.py:180] Training model SGDClassifier (iter 1/50, 0.001 min) ...
INFO [matcher.py:136] Best propensity score match found:
INFO [matcher.py:137] Model: SGDClassifier
INFO [matcher.py:139] * alpha: 1.5074398973827774
INFO [matcher.py:139] * class_weight: None
INFO [matcher.py:139] * early_stopping: True
INFO [matcher.py:139] * fit_intercept: True
INFO [matcher.py:139] * loss: log_loss
INFO [matcher.py:139] * max_iter: 1500
INFO [matcher.py:139] * penalty: l2
INFO [matcher.py:140] Score (beta): 0.0525
INFO [matcher.py:141] Solution time: 0.002 min
INFO [matcher.py:180] Training model LogisticRegression (iter 2/50, 0.002 min) ...
INFO [matcher.py:136] Best propensity score match found:
INFO [matcher.py:137] Model: LogisticRegression
INFO [matcher.py:139] * C: 0.05835496346821344
INFO [matcher.py:139] * fit_intercept: True
INFO [matcher.py:139] * max_iter: 500
INFO [matcher.py:139] * penalty: l2
INFO [matcher.py:139] * solver: saga
INFO [matcher.py:140] Score (beta): 0.0291
INFO [matcher.py:141] Solution time: 0.004 min
INFO [matcher.py:180] Training model LogisticRegression (iter 3/50, 0.004 min) ...
/Users/sprivite/src/pybalance/venv/pybalance/lib/python3.9/site-packages/sklearn/linear_model/_sag.py:350: ConvergenceWarning: The max_iter was reached which means the coef_ did not converge
warnings.warn(
INFO [matcher.py:180] Training model SGDClassifier (iter 4/50, 0.028 min) ...
INFO [matcher.py:180] Training model LogisticRegression (iter 5/50, 0.029 min) ...
/Users/sprivite/src/pybalance/venv/pybalance/lib/python3.9/site-packages/sklearn/linear_model/_sag.py:350: ConvergenceWarning: The max_iter was reached which means the coef_ did not converge
warnings.warn(
INFO [matcher.py:180] Training model LogisticRegression (iter 6/50, 0.050 min) ...
INFO [matcher.py:180] Training model LogisticRegression (iter 7/50, 0.052 min) ...
INFO [matcher.py:180] Training model SGDClassifier (iter 8/50, 0.055 min) ...
INFO [matcher.py:180] Training model LogisticRegression (iter 9/50, 0.056 min) ...
INFO [matcher.py:180] Training model LogisticRegression (iter 10/50, 0.073 min) ...
INFO [matcher.py:180] Training model LogisticRegression (iter 11/50, 0.075 min) ...
INFO [matcher.py:180] Training model SGDClassifier (iter 12/50, 0.078 min) ...
INFO [matcher.py:180] Training model SGDClassifier (iter 13/50, 0.079 min) ...
INFO [matcher.py:180] Training model SGDClassifier (iter 14/50, 0.080 min) ...
INFO [matcher.py:180] Training model SGDClassifier (iter 15/50, 0.082 min) ...
INFO [matcher.py:180] Training model SGDClassifier (iter 16/50, 0.083 min) ...
INFO [matcher.py:180] Training model SGDClassifier (iter 17/50, 0.085 min) ...
INFO [matcher.py:180] Training model LogisticRegression (iter 18/50, 0.087 min) ...
INFO [matcher.py:136] Best propensity score match found:
INFO [matcher.py:137] Model: LogisticRegression
INFO [matcher.py:139] * C: 2.3905570899706423
INFO [matcher.py:139] * fit_intercept: True
INFO [matcher.py:139] * max_iter: 500
INFO [matcher.py:139] * penalty: l2
INFO [matcher.py:139] * solver: saga
INFO [matcher.py:140] Score (beta): 0.0289
INFO [matcher.py:141] Solution time: 0.090 min
INFO [matcher.py:180] Training model LogisticRegression (iter 19/50, 0.090 min) ...
INFO [matcher.py:180] Training model SGDClassifier (iter 20/50, 0.101 min) ...
INFO [matcher.py:180] Training model SGDClassifier (iter 21/50, 0.102 min) ...
INFO [matcher.py:180] Training model SGDClassifier (iter 22/50, 0.104 min) ...
INFO [matcher.py:180] Training model SGDClassifier (iter 23/50, 0.105 min) ...
INFO [matcher.py:180] Training model LogisticRegression (iter 24/50, 0.107 min) ...
INFO [matcher.py:180] Training model SGDClassifier (iter 25/50, 0.109 min) ...
INFO [matcher.py:180] Training model LogisticRegression (iter 26/50, 0.111 min) ...
INFO [matcher.py:136] Best propensity score match found:
INFO [matcher.py:137] Model: LogisticRegression
INFO [matcher.py:139] * C: 0.2699411413616818
INFO [matcher.py:139] * fit_intercept: True
INFO [matcher.py:139] * max_iter: 500
INFO [matcher.py:139] * penalty: l1
INFO [matcher.py:139] * solver: saga
INFO [matcher.py:140] Score (beta): 0.0288
INFO [matcher.py:141] Solution time: 0.113 min
INFO [matcher.py:180] Training model LogisticRegression (iter 27/50, 0.113 min) ...
INFO [matcher.py:180] Training model LogisticRegression (iter 28/50, 0.115 min) ...
INFO [matcher.py:180] Training model SGDClassifier (iter 29/50, 0.133 min) ...
INFO [matcher.py:180] Training model LogisticRegression (iter 30/50, 0.135 min) ...
INFO [matcher.py:180] Training model SGDClassifier (iter 31/50, 0.137 min) ...
INFO [matcher.py:180] Training model LogisticRegression (iter 32/50, 0.139 min) ...
INFO [matcher.py:180] Training model SGDClassifier (iter 33/50, 0.141 min) ...
INFO [matcher.py:180] Training model SGDClassifier (iter 34/50, 0.142 min) ...
INFO [matcher.py:180] Training model LogisticRegression (iter 35/50, 0.144 min) ...
/Users/sprivite/src/pybalance/venv/pybalance/lib/python3.9/site-packages/sklearn/linear_model/_sag.py:350: ConvergenceWarning: The max_iter was reached which means the coef_ did not converge
warnings.warn(
INFO [matcher.py:180] Training model LogisticRegression (iter 36/50, 0.167 min) ...
INFO [matcher.py:180] Training model LogisticRegression (iter 37/50, 0.172 min) ...
/Users/sprivite/src/pybalance/venv/pybalance/lib/python3.9/site-packages/sklearn/linear_model/_sag.py:350: ConvergenceWarning: The max_iter was reached which means the coef_ did not converge
warnings.warn(
INFO [matcher.py:136] Best propensity score match found:
INFO [matcher.py:137] Model: LogisticRegression
INFO [matcher.py:139] * C: 0.5868985298319505
INFO [matcher.py:139] * fit_intercept: False
INFO [matcher.py:139] * max_iter: 500
INFO [matcher.py:139] * penalty: l1
INFO [matcher.py:139] * solver: saga
INFO [matcher.py:140] Score (beta): 0.0249
INFO [matcher.py:141] Solution time: 0.195 min
INFO [matcher.py:180] Training model SGDClassifier (iter 38/50, 0.195 min) ...
INFO [matcher.py:180] Training model SGDClassifier (iter 39/50, 0.197 min) ...
INFO [matcher.py:180] Training model LogisticRegression (iter 40/50, 0.199 min) ...
INFO [matcher.py:180] Training model SGDClassifier (iter 41/50, 0.201 min) ...
INFO [matcher.py:180] Training model LogisticRegression (iter 42/50, 0.203 min) ...
INFO [matcher.py:180] Training model LogisticRegression (iter 43/50, 0.213 min) ...
INFO [matcher.py:180] Training model SGDClassifier (iter 44/50, 0.233 min) ...
INFO [matcher.py:180] Training model SGDClassifier (iter 45/50, 0.234 min) ...
INFO [matcher.py:180] Training model LogisticRegression (iter 46/50, 0.235 min) ...
/Users/sprivite/src/pybalance/venv/pybalance/lib/python3.9/site-packages/sklearn/linear_model/_sag.py:350: ConvergenceWarning: The max_iter was reached which means the coef_ did not converge
warnings.warn(
INFO [matcher.py:180] Training model LogisticRegression (iter 47/50, 0.257 min) ...
/Users/sprivite/src/pybalance/venv/pybalance/lib/python3.9/site-packages/sklearn/linear_model/_sag.py:350: ConvergenceWarning: The max_iter was reached which means the coef_ did not converge
warnings.warn(
INFO [matcher.py:180] Training model SGDClassifier (iter 48/50, 0.279 min) ...
INFO [matcher.py:180] Training model SGDClassifier (iter 49/50, 0.281 min) ...
INFO [matcher.py:180] Training model LogisticRegression (iter 50/50, 0.282 min) ...
INFO [matcher.py:136] Best propensity score match found:
INFO [matcher.py:137] Model: LogisticRegression
INFO [matcher.py:139] * C: 0.5868985298319505
INFO [matcher.py:139] * fit_intercept: False
INFO [matcher.py:139] * max_iter: 500
INFO [matcher.py:139] * penalty: l1
INFO [matcher.py:139] * solver: saga
INFO [matcher.py:140] Score (beta): 0.0249
INFO [matcher.py:141] Solution time: 0.195 min
INFO [initialization.py:66] beta: 0.025
INFO [initialization.py:71] Included in initial population.
INFO [initialization.py:132] Sampling 512 candidate populations according to PROPENSITY distribution ...
INFO [initialization.py:132] Sampling 511 candidate populations according to UNIFORM distribution ...
INFO [logger.py:34] Generation 0
INFO [logger.py:35] remaining patients: 10000
INFO [logger.py:36] elapsed time: 0.31 min
INFO [logger.py:45] best beta: 0.02494 worst beta: 0.25118
INFO [logger.py:34] Generation 1000
INFO [logger.py:35] remaining patients: 7451
INFO [logger.py:36] elapsed time: 4.36 min
INFO [logger.py:45] best beta: 0.01141 worst beta: 0.24623
INFO [matcher.py:211] Time limit exceeded. Stopping.
[4]:
Headers Numeric:
['age', 'height', 'weight']
Headers Categoric:
['gender', 'haircolor', 'country', 'binary_0', 'binary_1', 'binary_2', 'binary_3']
Populations
['pool', 'target']
['age', 'height', 'weight']
Headers Categoric:
['gender', 'haircolor', 'country', 'binary_0', 'binary_1', 'binary_2', 'binary_3']
Populations
['pool', 'target']
age | height | weight | gender | haircolor | country | population | binary_0 | binary_1 | binary_2 | binary_3 | patient_id | |
---|---|---|---|---|---|---|---|---|---|---|---|---|
0 | 55.261578 | 139.396134 | 94.438359 | 0.0 | 2 | 2 | target | 0 | 0 | 1 | 1 | 10000 |
1 | 63.113091 | 165.563337 | 67.433016 | 1.0 | 2 | 2 | target | 0 | 1 | 1 | 0 | 10001 |
2 | 58.232216 | 160.859857 | 71.915385 | 1.0 | 0 | 2 | target | 0 | 0 | 0 | 0 | 10002 |
3 | 58.996941 | 140.357415 | 115.606615 | 1.0 | 0 | 3 | target | 1 | 1 | 0 | 0 | 10003 |
4 | 36.850195 | 189.983706 | 53.000581 | 0.0 | 2 | 5 | target | 0 | 0 | 0 | 0 | 10004 |
... | ... | ... | ... | ... | ... | ... | ... | ... | ... | ... | ... | ... |
43 | 28.382714 | 155.203257 | 73.126127 | 0.0 | 2 | 5 | pool | 1 | 1 | 0 | 1 | 43 |
4050 | 44.186246 | 133.221816 | 97.047691 | 1.0 | 0 | 4 | pool | 0 | 0 | 0 | 1 | 4050 |
8313 | 46.510049 | 130.960298 | 88.501597 | 1.0 | 2 | 4 | pool | 0 | 0 | 1 | 0 | 8313 |
7166 | 57.061793 | 157.544063 | 70.784043 | 1.0 | 2 | 4 | pool | 0 | 1 | 0 | 1 | 7166 |
569 | 50.174501 | 161.973210 | 83.131287 | 1.0 | 1 | 1 | pool | 0 | 0 | 0 | 0 | 569 |
2000 rows × 12 columns
[5]:
%matplotlib inline
match = matcher_beta.get_best_match()
m_data = m.copy().get_population('pool')
m_data.loc[:, 'population'] = m_data['population'] + ' (prematch)'
match.append(m_data)
fig = plot_per_feature_loss(match, beta, 'target', debin=False)
fig = plot_numeric_features(match, hue_order=['pool (prematch)', 'pool', 'target', ])
fig = plot_categoric_features(match, hue_order=['pool (prematch)', 'pool', 'target'])
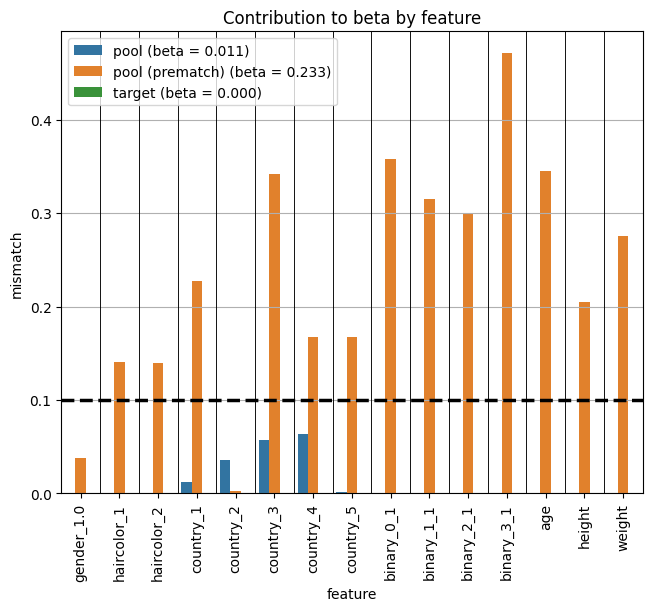
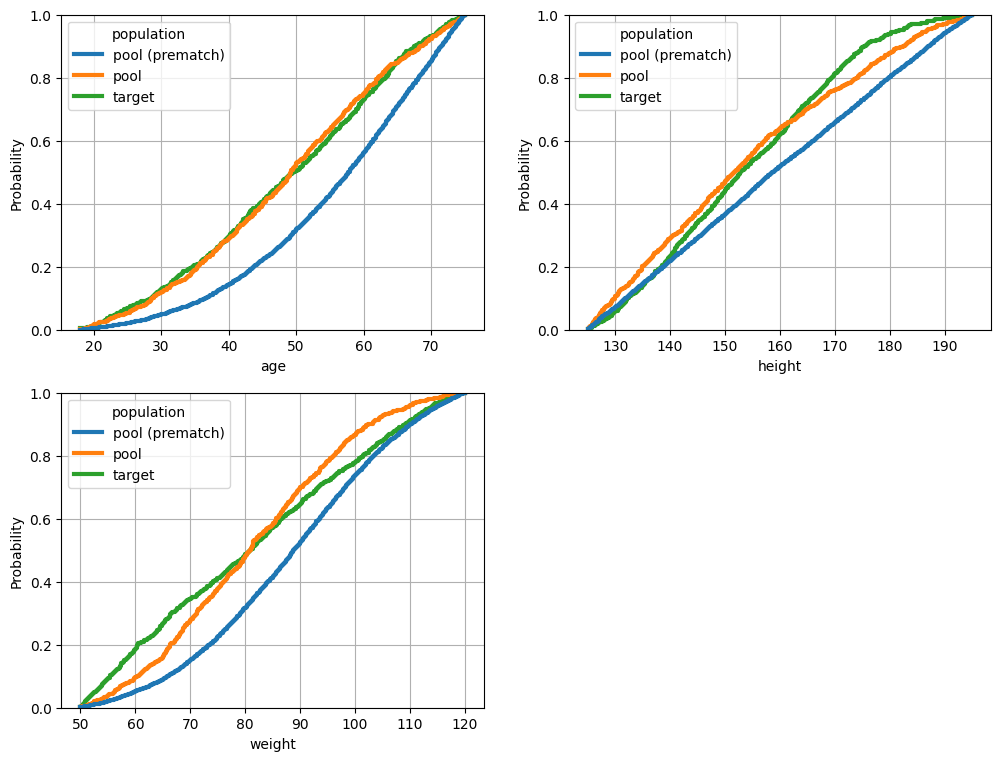
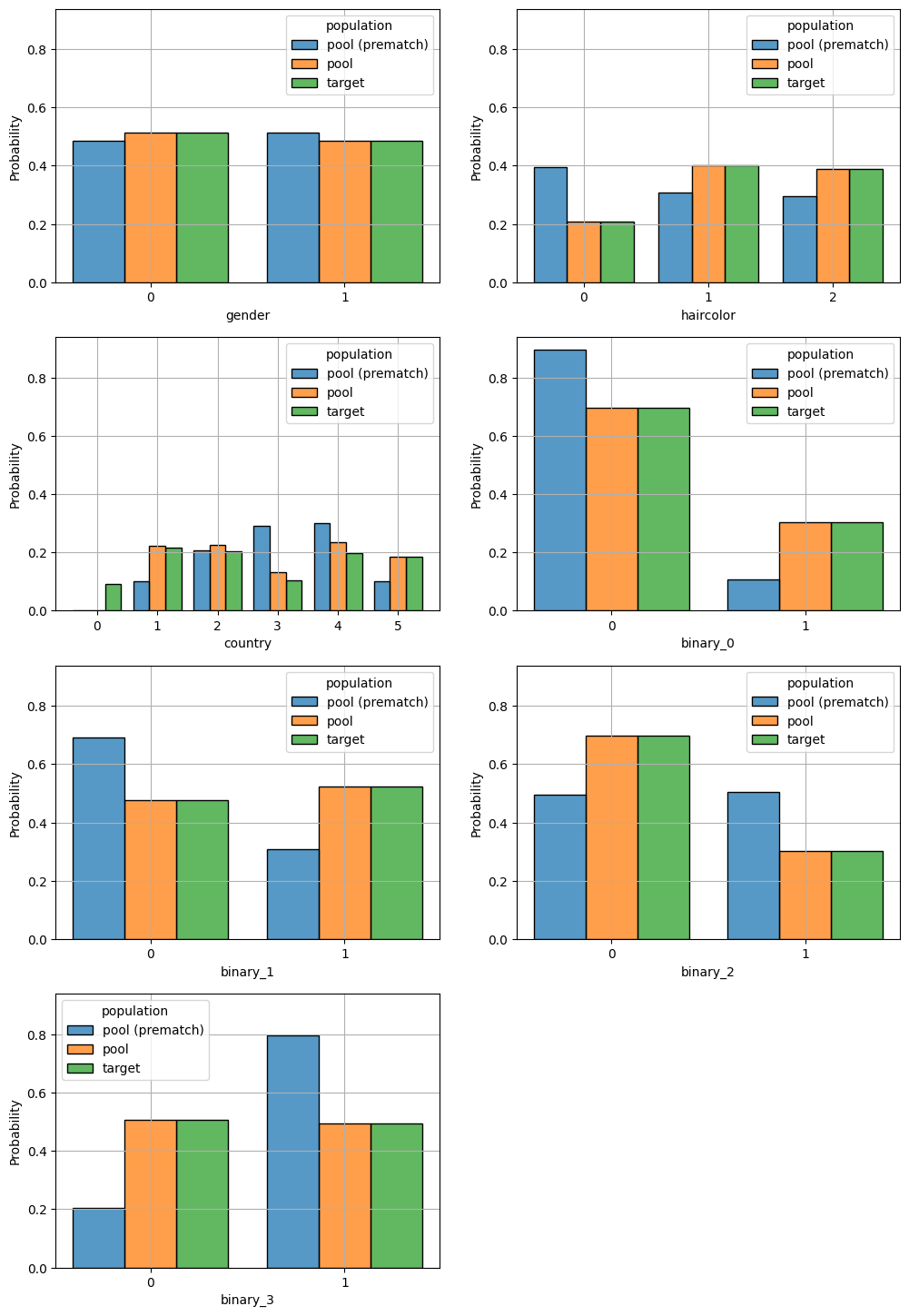
Optimize Beta^2
[6]:
objective = beta2 = BetaSquaredBalance(m)
matcher = matcher_beta2 = GeneticMatcher(
matching_data = m,
objective = objective,
log_every = 1000,
n_generations = 5000,
time_limit = time_limit
)
matcher.get_params()
INFO [matcher.py:125] cpu
[6]:
{'objective': 'beta_squared',
'candidate_population_size': 1000,
'n_candidate_populations': 1024,
'n_keep_best': 256,
'n_voting_populations': 256,
'n_mutation': 256,
'n_generations': 5000,
'n_iter_no_change': 100,
'time_limit': 300,
'max_batch_size_gb': 2,
'seed': 1234,
'verbose': True,
'log_every': 1000,
'initialization': {'benchmarks': {'propensity': 'include'},
'sampling': {'propensity': 1.0, 'uniform': 1.0}}}
[7]:
match = matcher.match()
INFO [initialization.py:31] Optimizing balance with genetic algorithm ...
INFO [initialization.py:32] Initial balance scores:
INFO [initialization.py:37] beta_squared: 0.263
INFO [initialization.py:38] Initializing candidate populations ...
INFO [initialization.py:86] Computing PROPENSITY 1-1 matching method ...
INFO [matcher.py:180] Training model SGDClassifier (iter 1/50, 0.001 min) ...
INFO [matcher.py:136] Best propensity score match found:
INFO [matcher.py:137] Model: SGDClassifier
INFO [matcher.py:139] * alpha: 1.5074398973827774
INFO [matcher.py:139] * class_weight: None
INFO [matcher.py:139] * early_stopping: True
INFO [matcher.py:139] * fit_intercept: True
INFO [matcher.py:139] * loss: log_loss
INFO [matcher.py:139] * max_iter: 1500
INFO [matcher.py:139] * penalty: l2
INFO [matcher.py:140] Score (beta_squared): 0.0603
INFO [matcher.py:141] Solution time: 0.004 min
INFO [matcher.py:180] Training model LogisticRegression (iter 2/50, 0.004 min) ...
INFO [matcher.py:136] Best propensity score match found:
INFO [matcher.py:137] Model: LogisticRegression
INFO [matcher.py:139] * C: 0.05835496346821344
INFO [matcher.py:139] * fit_intercept: True
INFO [matcher.py:139] * max_iter: 500
INFO [matcher.py:139] * penalty: l2
INFO [matcher.py:139] * solver: saga
INFO [matcher.py:140] Score (beta_squared): 0.0374
INFO [matcher.py:141] Solution time: 0.007 min
INFO [matcher.py:180] Training model LogisticRegression (iter 3/50, 0.007 min) ...
/Users/sprivite/src/pybalance/venv/pybalance/lib/python3.9/site-packages/sklearn/linear_model/_sag.py:350: ConvergenceWarning: The max_iter was reached which means the coef_ did not converge
warnings.warn(
INFO [matcher.py:136] Best propensity score match found:
INFO [matcher.py:137] Model: LogisticRegression
INFO [matcher.py:139] * C: 16.16555309446666
INFO [matcher.py:139] * fit_intercept: False
INFO [matcher.py:139] * max_iter: 500
INFO [matcher.py:139] * penalty: l1
INFO [matcher.py:139] * solver: saga
INFO [matcher.py:140] Score (beta_squared): 0.0357
INFO [matcher.py:141] Solution time: 0.044 min
INFO [matcher.py:180] Training model SGDClassifier (iter 4/50, 0.044 min) ...
INFO [matcher.py:180] Training model LogisticRegression (iter 5/50, 0.048 min) ...
/Users/sprivite/src/pybalance/venv/pybalance/lib/python3.9/site-packages/sklearn/linear_model/_sag.py:350: ConvergenceWarning: The max_iter was reached which means the coef_ did not converge
warnings.warn(
INFO [matcher.py:136] Best propensity score match found:
INFO [matcher.py:137] Model: LogisticRegression
INFO [matcher.py:139] * C: 54.02072493419677
INFO [matcher.py:139] * fit_intercept: False
INFO [matcher.py:139] * max_iter: 500
INFO [matcher.py:139] * penalty: l2
INFO [matcher.py:139] * solver: saga
INFO [matcher.py:140] Score (beta_squared): 0.0347
INFO [matcher.py:141] Solution time: 0.080 min
INFO [matcher.py:180] Training model LogisticRegression (iter 6/50, 0.081 min) ...
INFO [matcher.py:180] Training model LogisticRegression (iter 7/50, 0.085 min) ...
INFO [matcher.py:180] Training model SGDClassifier (iter 8/50, 0.089 min) ...
INFO [matcher.py:180] Training model LogisticRegression (iter 9/50, 0.092 min) ...
INFO [matcher.py:180] Training model LogisticRegression (iter 10/50, 0.117 min) ...
INFO [matcher.py:180] Training model LogisticRegression (iter 11/50, 0.120 min) ...
INFO [matcher.py:180] Training model SGDClassifier (iter 12/50, 0.124 min) ...
INFO [matcher.py:180] Training model SGDClassifier (iter 13/50, 0.126 min) ...
INFO [matcher.py:180] Training model SGDClassifier (iter 14/50, 0.129 min) ...
INFO [matcher.py:180] Training model SGDClassifier (iter 15/50, 0.131 min) ...
INFO [matcher.py:180] Training model SGDClassifier (iter 16/50, 0.134 min) ...
INFO [matcher.py:180] Training model SGDClassifier (iter 17/50, 0.136 min) ...
INFO [matcher.py:180] Training model LogisticRegression (iter 18/50, 0.139 min) ...
INFO [matcher.py:180] Training model LogisticRegression (iter 19/50, 0.143 min) ...
INFO [matcher.py:180] Training model SGDClassifier (iter 20/50, 0.165 min) ...
INFO [matcher.py:180] Training model SGDClassifier (iter 21/50, 0.168 min) ...
INFO [matcher.py:180] Training model SGDClassifier (iter 22/50, 0.170 min) ...
INFO [matcher.py:180] Training model SGDClassifier (iter 23/50, 0.177 min) ...
INFO [matcher.py:180] Training model LogisticRegression (iter 24/50, 0.181 min) ...
INFO [matcher.py:180] Training model SGDClassifier (iter 25/50, 0.186 min) ...
INFO [matcher.py:180] Training model LogisticRegression (iter 26/50, 0.188 min) ...
INFO [matcher.py:180] Training model LogisticRegression (iter 27/50, 0.192 min) ...
INFO [matcher.py:180] Training model LogisticRegression (iter 28/50, 0.196 min) ...
INFO [matcher.py:180] Training model SGDClassifier (iter 29/50, 0.224 min) ...
INFO [matcher.py:180] Training model LogisticRegression (iter 30/50, 0.226 min) ...
INFO [matcher.py:180] Training model SGDClassifier (iter 31/50, 0.232 min) ...
INFO [matcher.py:180] Training model LogisticRegression (iter 32/50, 0.235 min) ...
INFO [matcher.py:180] Training model SGDClassifier (iter 33/50, 0.238 min) ...
INFO [matcher.py:180] Training model SGDClassifier (iter 34/50, 0.241 min) ...
INFO [matcher.py:180] Training model LogisticRegression (iter 35/50, 0.245 min) ...
/Users/sprivite/src/pybalance/venv/pybalance/lib/python3.9/site-packages/sklearn/linear_model/_sag.py:350: ConvergenceWarning: The max_iter was reached which means the coef_ did not converge
warnings.warn(
INFO [matcher.py:180] Training model LogisticRegression (iter 36/50, 0.280 min) ...
INFO [matcher.py:180] Training model LogisticRegression (iter 37/50, 0.288 min) ...
/Users/sprivite/src/pybalance/venv/pybalance/lib/python3.9/site-packages/sklearn/linear_model/_sag.py:350: ConvergenceWarning: The max_iter was reached which means the coef_ did not converge
warnings.warn(
INFO [matcher.py:136] Best propensity score match found:
INFO [matcher.py:137] Model: LogisticRegression
INFO [matcher.py:139] * C: 0.5868985298319505
INFO [matcher.py:139] * fit_intercept: False
INFO [matcher.py:139] * max_iter: 500
INFO [matcher.py:139] * penalty: l1
INFO [matcher.py:139] * solver: saga
INFO [matcher.py:140] Score (beta_squared): 0.0311
INFO [matcher.py:141] Solution time: 0.322 min
INFO [matcher.py:180] Training model SGDClassifier (iter 38/50, 0.323 min) ...
INFO [matcher.py:180] Training model SGDClassifier (iter 39/50, 0.325 min) ...
INFO [matcher.py:180] Training model LogisticRegression (iter 40/50, 0.328 min) ...
INFO [matcher.py:180] Training model SGDClassifier (iter 41/50, 0.331 min) ...
INFO [matcher.py:180] Training model LogisticRegression (iter 42/50, 0.333 min) ...
INFO [matcher.py:180] Training model LogisticRegression (iter 43/50, 0.350 min) ...
INFO [matcher.py:180] Training model SGDClassifier (iter 44/50, 0.381 min) ...
INFO [matcher.py:180] Training model SGDClassifier (iter 45/50, 0.385 min) ...
INFO [matcher.py:180] Training model LogisticRegression (iter 46/50, 0.387 min) ...
/Users/sprivite/src/pybalance/venv/pybalance/lib/python3.9/site-packages/sklearn/linear_model/_sag.py:350: ConvergenceWarning: The max_iter was reached which means the coef_ did not converge
warnings.warn(
INFO [matcher.py:180] Training model LogisticRegression (iter 47/50, 0.423 min) ...
/Users/sprivite/src/pybalance/venv/pybalance/lib/python3.9/site-packages/sklearn/linear_model/_sag.py:350: ConvergenceWarning: The max_iter was reached which means the coef_ did not converge
warnings.warn(
INFO [matcher.py:180] Training model SGDClassifier (iter 48/50, 0.458 min) ...
INFO [matcher.py:180] Training model SGDClassifier (iter 49/50, 0.460 min) ...
INFO [matcher.py:180] Training model LogisticRegression (iter 50/50, 0.462 min) ...
INFO [matcher.py:136] Best propensity score match found:
INFO [matcher.py:137] Model: LogisticRegression
INFO [matcher.py:139] * C: 0.5868985298319505
INFO [matcher.py:139] * fit_intercept: False
INFO [matcher.py:139] * max_iter: 500
INFO [matcher.py:139] * penalty: l1
INFO [matcher.py:139] * solver: saga
INFO [matcher.py:140] Score (beta_squared): 0.0311
INFO [matcher.py:141] Solution time: 0.322 min
INFO [initialization.py:66] beta_squared: 0.031
INFO [initialization.py:71] Included in initial population.
INFO [initialization.py:132] Sampling 512 candidate populations according to PROPENSITY distribution ...
INFO [initialization.py:132] Sampling 511 candidate populations according to UNIFORM distribution ...
INFO [logger.py:34] Generation 0
INFO [logger.py:35] remaining patients: 10000
INFO [logger.py:36] elapsed time: 0.51 min
INFO [logger.py:45] best beta_squared: 0.03106 worst beta_squared: 0.28163
INFO [matcher.py:211] Time limit exceeded. Stopping.
[8]:
%matplotlib inline
match = matcher_beta2.get_best_match()
m_data = m.copy().get_population('pool')
m_data.loc[:, 'population'] = m_data['population'] + ' (prematch)'
match.append(m_data)
fig = plot_per_feature_loss(match, beta, 'target', debin=False)
fig = plot_numeric_features(match, hue_order=['pool (prematch)', 'pool', 'target', ])
fig = plot_categoric_features(match, hue_order=['pool (prematch)', 'pool', 'target'])
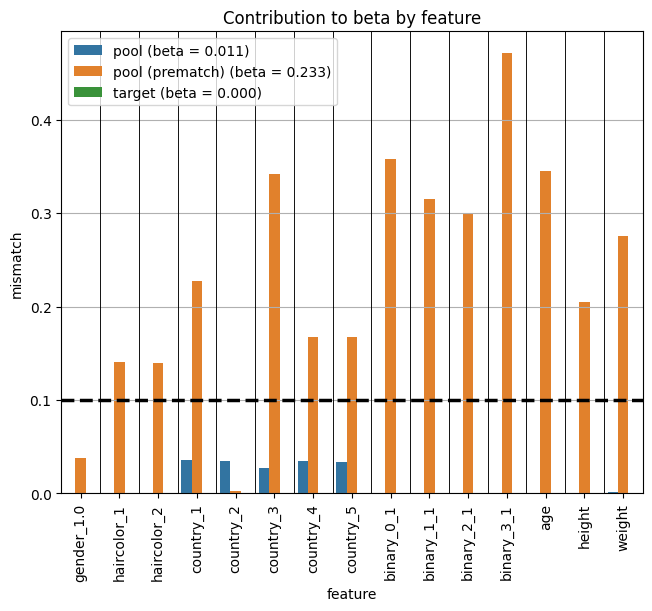
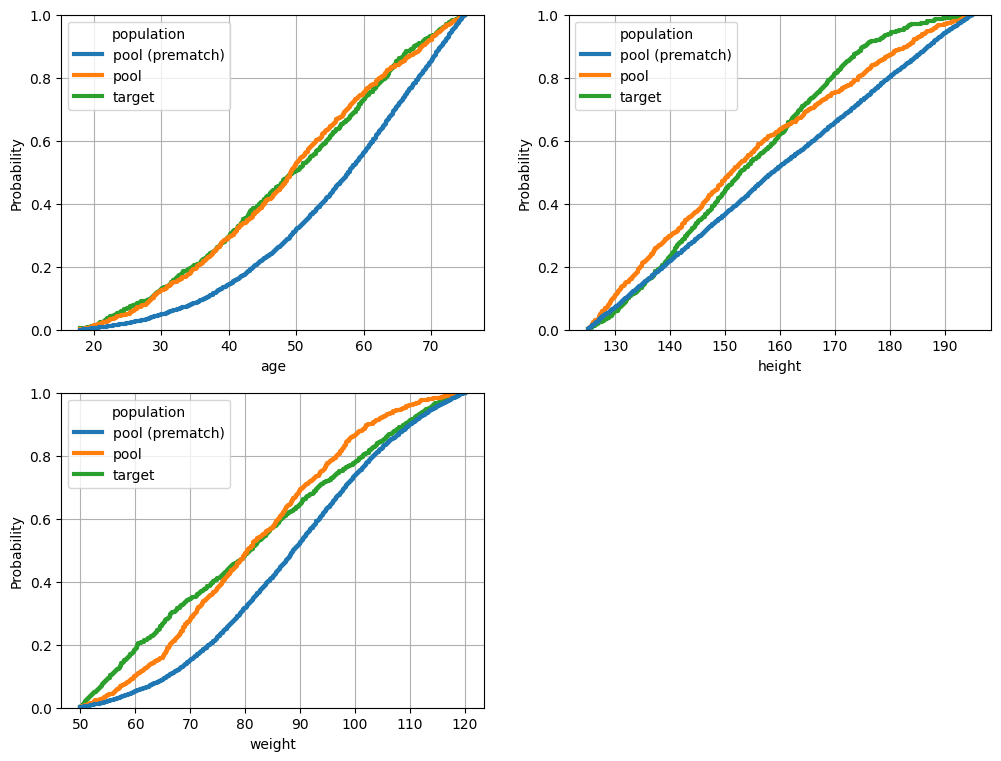
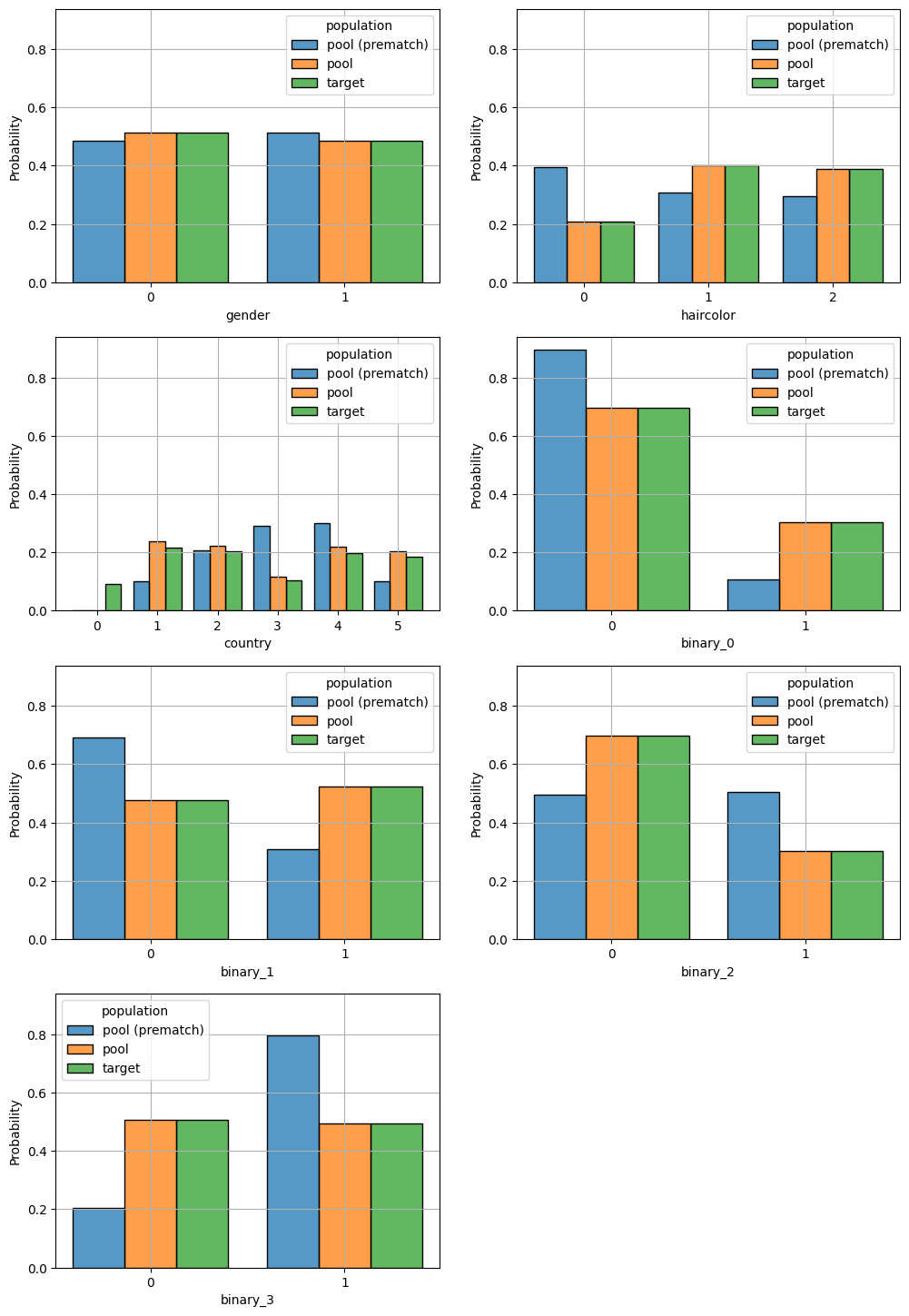
Optimize Gamma (Area Between CDFs)
[9]:
objective = gamma = GammaBalance(m, feature_weights={'age':2})
matcher = matcher_gamma = GeneticMatcher(
matching_data = m,
objective = objective,
log_every = 1000,
n_generations = 5000,
time_limit = time_limit
)
matcher.get_params()
INFO [preprocess.py:335] Discretized age with bins [18.05, 27.54, 37.04, 46.53, 56.02, 65.51, 75.0].
INFO [preprocess.py:335] Discretized height with bins [125.01, 136.68, 148.34, 160.01, 171.67, 183.34, 195.0].
INFO [preprocess.py:335] Discretized weight with bins [50.0, 61.67, 73.33, 85.0, 96.66, 108.33, 120.0].
INFO [matcher.py:125] cpu
[9]:
{'objective': 'gamma',
'candidate_population_size': 1000,
'n_candidate_populations': 1024,
'n_keep_best': 256,
'n_voting_populations': 256,
'n_mutation': 256,
'n_generations': 5000,
'n_iter_no_change': 100,
'time_limit': 300,
'max_batch_size_gb': 2,
'seed': 1234,
'verbose': True,
'log_every': 1000,
'initialization': {'benchmarks': {'propensity': 'include'},
'sampling': {'propensity': 1.0, 'uniform': 1.0}}}
[10]:
match = matcher.match()
INFO [initialization.py:31] Optimizing balance with genetic algorithm ...
INFO [initialization.py:32] Initial balance scores:
INFO [initialization.py:37] gamma: 0.217
INFO [initialization.py:38] Initializing candidate populations ...
INFO [initialization.py:86] Computing PROPENSITY 1-1 matching method ...
INFO [matcher.py:180] Training model SGDClassifier (iter 1/50, 0.001 min) ...
INFO [matcher.py:136] Best propensity score match found:
INFO [matcher.py:137] Model: SGDClassifier
INFO [matcher.py:139] * alpha: 1.5074398973827774
INFO [matcher.py:139] * class_weight: None
INFO [matcher.py:139] * early_stopping: True
INFO [matcher.py:139] * fit_intercept: True
INFO [matcher.py:139] * loss: log_loss
INFO [matcher.py:139] * max_iter: 1500
INFO [matcher.py:139] * penalty: l2
INFO [matcher.py:140] Score (gamma): 0.1083
INFO [matcher.py:141] Solution time: 0.004 min
INFO [matcher.py:180] Training model LogisticRegression (iter 2/50, 0.004 min) ...
INFO [matcher.py:136] Best propensity score match found:
INFO [matcher.py:137] Model: LogisticRegression
INFO [matcher.py:139] * C: 0.05835496346821344
INFO [matcher.py:139] * fit_intercept: True
INFO [matcher.py:139] * max_iter: 500
INFO [matcher.py:139] * penalty: l2
INFO [matcher.py:139] * solver: saga
INFO [matcher.py:140] Score (gamma): 0.0391
INFO [matcher.py:141] Solution time: 0.008 min
INFO [matcher.py:180] Training model LogisticRegression (iter 3/50, 0.008 min) ...
/Users/sprivite/src/pybalance/venv/pybalance/lib/python3.9/site-packages/sklearn/linear_model/_sag.py:350: ConvergenceWarning: The max_iter was reached which means the coef_ did not converge
warnings.warn(
INFO [matcher.py:136] Best propensity score match found:
INFO [matcher.py:137] Model: LogisticRegression
INFO [matcher.py:139] * C: 16.16555309446666
INFO [matcher.py:139] * fit_intercept: False
INFO [matcher.py:139] * max_iter: 500
INFO [matcher.py:139] * penalty: l1
INFO [matcher.py:139] * solver: saga
INFO [matcher.py:140] Score (gamma): 0.0376
INFO [matcher.py:141] Solution time: 0.055 min
INFO [matcher.py:180] Training model SGDClassifier (iter 4/50, 0.055 min) ...
INFO [matcher.py:180] Training model LogisticRegression (iter 5/50, 0.058 min) ...
/Users/sprivite/src/pybalance/venv/pybalance/lib/python3.9/site-packages/sklearn/linear_model/_sag.py:350: ConvergenceWarning: The max_iter was reached which means the coef_ did not converge
warnings.warn(
INFO [matcher.py:136] Best propensity score match found:
INFO [matcher.py:137] Model: LogisticRegression
INFO [matcher.py:139] * C: 54.02072493419677
INFO [matcher.py:139] * fit_intercept: False
INFO [matcher.py:139] * max_iter: 500
INFO [matcher.py:139] * penalty: l2
INFO [matcher.py:139] * solver: saga
INFO [matcher.py:140] Score (gamma): 0.0337
INFO [matcher.py:141] Solution time: 0.091 min
INFO [matcher.py:180] Training model LogisticRegression (iter 6/50, 0.091 min) ...
INFO [matcher.py:180] Training model LogisticRegression (iter 7/50, 0.095 min) ...
INFO [matcher.py:180] Training model SGDClassifier (iter 8/50, 0.098 min) ...
INFO [matcher.py:180] Training model LogisticRegression (iter 9/50, 0.101 min) ...
/Users/sprivite/src/pybalance/venv/pybalance/lib/python3.9/site-packages/sklearn/linear_model/_sag.py:350: ConvergenceWarning: The max_iter was reached which means the coef_ did not converge
warnings.warn(
INFO [matcher.py:136] Best propensity score match found:
INFO [matcher.py:137] Model: LogisticRegression
INFO [matcher.py:139] * C: 13.179630432958701
INFO [matcher.py:139] * fit_intercept: False
INFO [matcher.py:139] * max_iter: 500
INFO [matcher.py:139] * penalty: l2
INFO [matcher.py:139] * solver: saga
INFO [matcher.py:140] Score (gamma): 0.0269
INFO [matcher.py:141] Solution time: 0.135 min
INFO [matcher.py:180] Training model LogisticRegression (iter 10/50, 0.135 min) ...
INFO [matcher.py:180] Training model LogisticRegression (iter 11/50, 0.138 min) ...
INFO [matcher.py:180] Training model SGDClassifier (iter 12/50, 0.142 min) ...
INFO [matcher.py:180] Training model SGDClassifier (iter 13/50, 0.144 min) ...
INFO [matcher.py:180] Training model SGDClassifier (iter 14/50, 0.146 min) ...
INFO [matcher.py:180] Training model SGDClassifier (iter 15/50, 0.149 min) ...
INFO [matcher.py:180] Training model SGDClassifier (iter 16/50, 0.151 min) ...
INFO [matcher.py:180] Training model SGDClassifier (iter 17/50, 0.154 min) ...
INFO [matcher.py:180] Training model LogisticRegression (iter 18/50, 0.158 min) ...
INFO [matcher.py:180] Training model LogisticRegression (iter 19/50, 0.165 min) ...
/Users/sprivite/src/pybalance/venv/pybalance/lib/python3.9/site-packages/sklearn/linear_model/_sag.py:350: ConvergenceWarning: The max_iter was reached which means the coef_ did not converge
warnings.warn(
INFO [matcher.py:180] Training model SGDClassifier (iter 20/50, 0.196 min) ...
INFO [matcher.py:180] Training model SGDClassifier (iter 21/50, 0.199 min) ...
INFO [matcher.py:180] Training model SGDClassifier (iter 22/50, 0.201 min) ...
INFO [matcher.py:180] Training model SGDClassifier (iter 23/50, 0.203 min) ...
INFO [matcher.py:180] Training model LogisticRegression (iter 24/50, 0.206 min) ...
INFO [matcher.py:180] Training model SGDClassifier (iter 25/50, 0.211 min) ...
INFO [matcher.py:180] Training model LogisticRegression (iter 26/50, 0.213 min) ...
INFO [matcher.py:180] Training model LogisticRegression (iter 27/50, 0.219 min) ...
INFO [matcher.py:180] Training model LogisticRegression (iter 28/50, 0.222 min) ...
/Users/sprivite/src/pybalance/venv/pybalance/lib/python3.9/site-packages/sklearn/linear_model/_sag.py:350: ConvergenceWarning: The max_iter was reached which means the coef_ did not converge
warnings.warn(
INFO [matcher.py:180] Training model SGDClassifier (iter 29/50, 0.263 min) ...
INFO [matcher.py:180] Training model LogisticRegression (iter 30/50, 0.265 min) ...
INFO [matcher.py:180] Training model SGDClassifier (iter 31/50, 0.271 min) ...
INFO [matcher.py:180] Training model LogisticRegression (iter 32/50, 0.273 min) ...
INFO [matcher.py:180] Training model SGDClassifier (iter 33/50, 0.277 min) ...
INFO [matcher.py:180] Training model SGDClassifier (iter 34/50, 0.279 min) ...
INFO [matcher.py:180] Training model LogisticRegression (iter 35/50, 0.281 min) ...
/Users/sprivite/src/pybalance/venv/pybalance/lib/python3.9/site-packages/sklearn/linear_model/_sag.py:350: ConvergenceWarning: The max_iter was reached which means the coef_ did not converge
warnings.warn(
INFO [matcher.py:180] Training model LogisticRegression (iter 36/50, 0.320 min) ...
INFO [matcher.py:180] Training model LogisticRegression (iter 37/50, 0.333 min) ...
/Users/sprivite/src/pybalance/venv/pybalance/lib/python3.9/site-packages/sklearn/linear_model/_sag.py:350: ConvergenceWarning: The max_iter was reached which means the coef_ did not converge
warnings.warn(
INFO [matcher.py:180] Training model SGDClassifier (iter 38/50, 0.370 min) ...
INFO [matcher.py:180] Training model SGDClassifier (iter 39/50, 0.372 min) ...
INFO [matcher.py:180] Training model LogisticRegression (iter 40/50, 0.374 min) ...
INFO [matcher.py:180] Training model SGDClassifier (iter 41/50, 0.378 min) ...
INFO [matcher.py:180] Training model LogisticRegression (iter 42/50, 0.380 min) ...
/Users/sprivite/src/pybalance/venv/pybalance/lib/python3.9/site-packages/sklearn/linear_model/_sag.py:350: ConvergenceWarning: The max_iter was reached which means the coef_ did not converge
warnings.warn(
INFO [matcher.py:180] Training model LogisticRegression (iter 43/50, 0.409 min) ...
/Users/sprivite/src/pybalance/venv/pybalance/lib/python3.9/site-packages/sklearn/linear_model/_sag.py:350: ConvergenceWarning: The max_iter was reached which means the coef_ did not converge
warnings.warn(
INFO [matcher.py:180] Training model SGDClassifier (iter 44/50, 0.446 min) ...
INFO [matcher.py:180] Training model SGDClassifier (iter 45/50, 0.448 min) ...
INFO [matcher.py:180] Training model LogisticRegression (iter 46/50, 0.450 min) ...
/Users/sprivite/src/pybalance/venv/pybalance/lib/python3.9/site-packages/sklearn/linear_model/_sag.py:350: ConvergenceWarning: The max_iter was reached which means the coef_ did not converge
warnings.warn(
INFO [matcher.py:180] Training model LogisticRegression (iter 47/50, 0.486 min) ...
/Users/sprivite/src/pybalance/venv/pybalance/lib/python3.9/site-packages/sklearn/linear_model/_sag.py:350: ConvergenceWarning: The max_iter was reached which means the coef_ did not converge
warnings.warn(
INFO [matcher.py:180] Training model SGDClassifier (iter 48/50, 0.524 min) ...
INFO [matcher.py:180] Training model SGDClassifier (iter 49/50, 0.526 min) ...
INFO [matcher.py:180] Training model LogisticRegression (iter 50/50, 0.528 min) ...
INFO [matcher.py:136] Best propensity score match found:
INFO [matcher.py:137] Model: LogisticRegression
INFO [matcher.py:139] * C: 13.179630432958701
INFO [matcher.py:139] * fit_intercept: False
INFO [matcher.py:139] * max_iter: 500
INFO [matcher.py:139] * penalty: l2
INFO [matcher.py:139] * solver: saga
INFO [matcher.py:140] Score (gamma): 0.0269
INFO [matcher.py:141] Solution time: 0.135 min
INFO [initialization.py:66] gamma: 0.027
INFO [initialization.py:71] Included in initial population.
INFO [initialization.py:132] Sampling 512 candidate populations according to PROPENSITY distribution ...
INFO [initialization.py:132] Sampling 511 candidate populations according to UNIFORM distribution ...
INFO [logger.py:34] Generation 0
INFO [logger.py:35] remaining patients: 10000
INFO [logger.py:36] elapsed time: 0.55 min
INFO [logger.py:45] best gamma: 0.02688 worst gamma: 0.23595
INFO [logger.py:34] Generation 1000
INFO [logger.py:35] remaining patients: 7375
INFO [logger.py:36] elapsed time: 4.93 min
INFO [logger.py:45] best gamma: 0.01151 worst gamma: 0.24080
INFO [matcher.py:211] Time limit exceeded. Stopping.
[11]:
%matplotlib inline
match = matcher.get_best_match()
m_data = m.copy().get_population('pool')
m_data.loc[:, 'population'] = m_data['population'] + ' (prematch)'
match.append(m_data)
fig = plot_per_feature_loss(match, gamma, 'target', debin=False)
fig = plot_numeric_features(match, hue_order=['pool (prematch)', 'pool', 'target', ])
fig = plot_categoric_features(match, hue_order=['pool (prematch)', 'pool', 'target'])
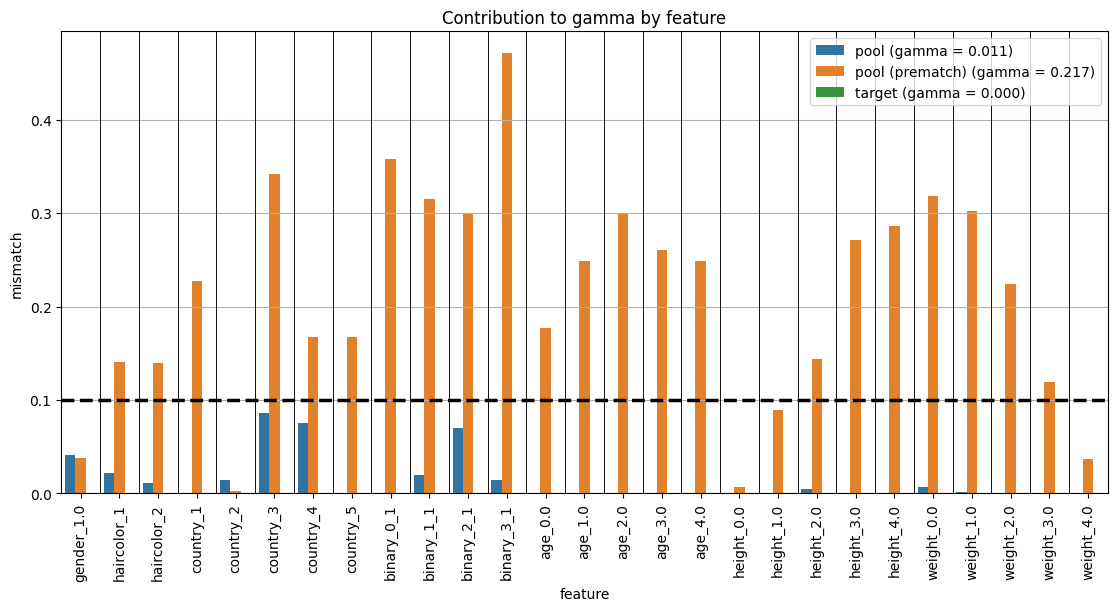
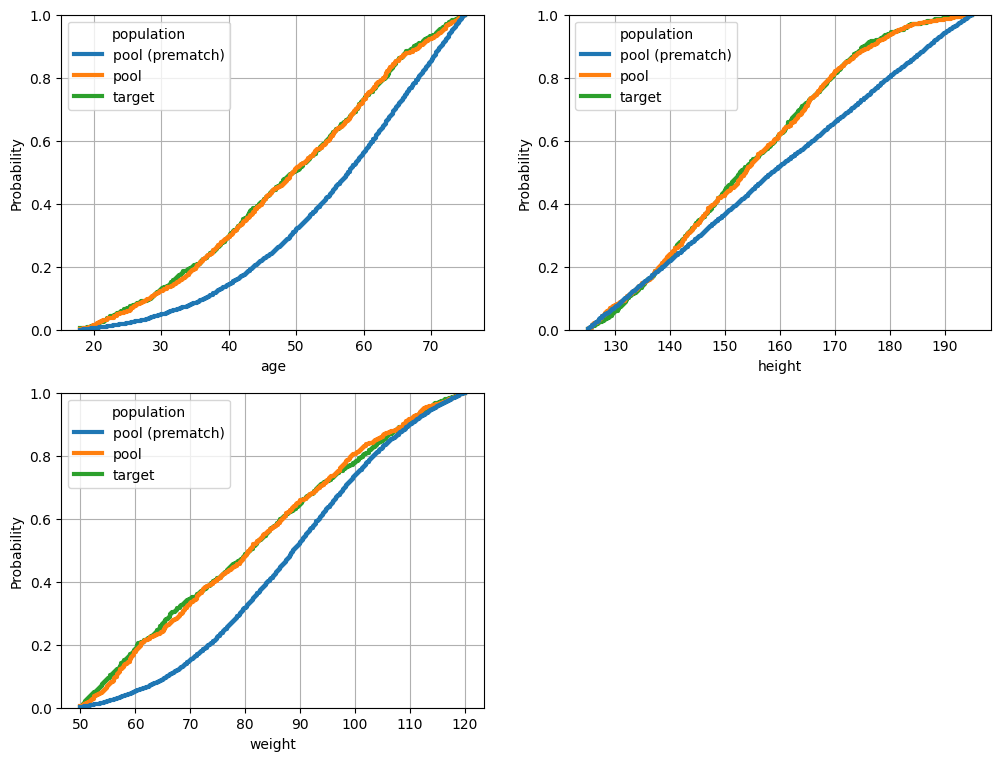
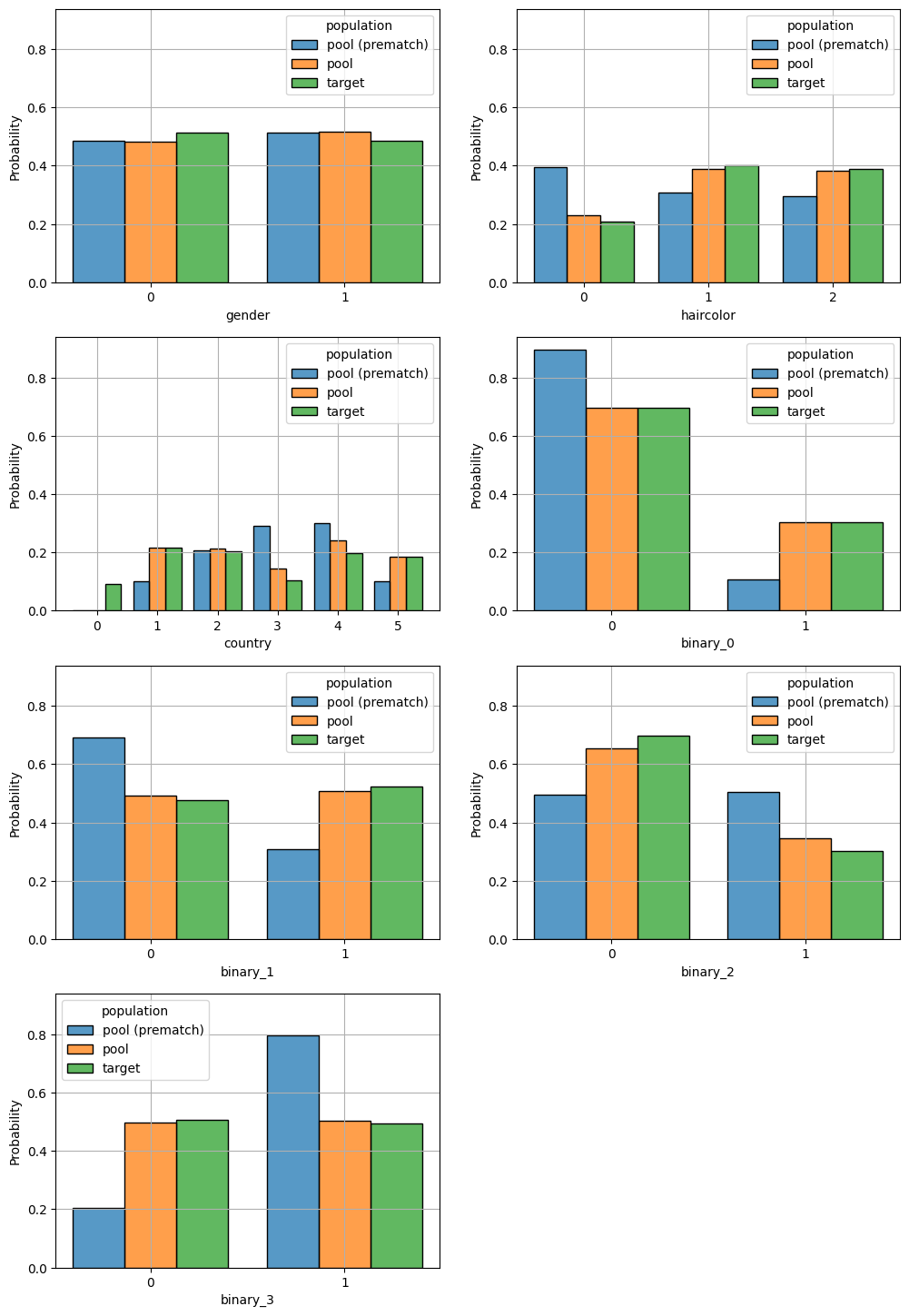